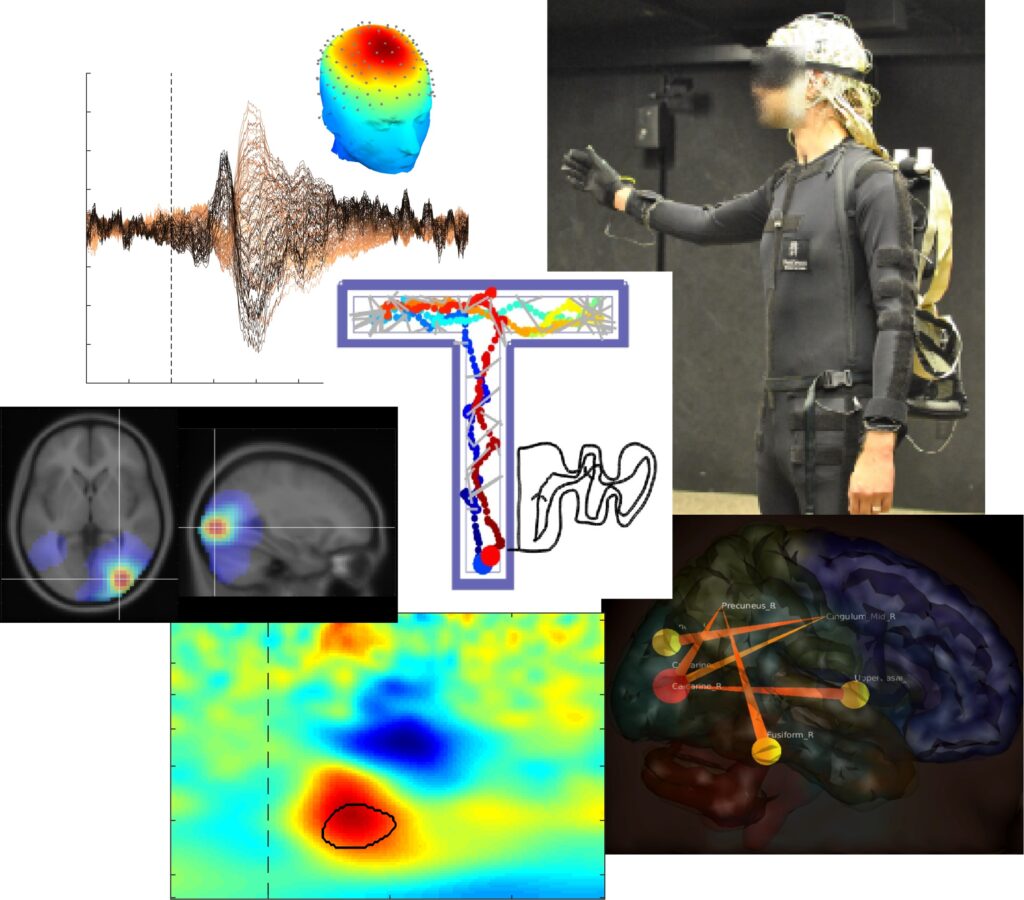
Journal page
https://onlinelibrary.wiley.com/doi/epdf/10.1111/ejn.15131
AudioMaze is Swartz Center’s main Mobile Brain-Body Imaging (MoBI) projects that was multi-million-dollar funded, and the dedicated lab has been in ‘the downstairs’ for more than a decade. Basically, the idea is that a blindfolded subject walks through the digitally defined mazes which has in programmable walls that returns audio feedback when touched, hence AudioMaze. Because blindfolded subjects need to walk very slowly, and the spatial probing is limited to stretching the right arm, subject’s behaviors becomes very slow, stereotypical, and repeated, all of which is suitable for event-related brain potential analysis.
The highlight of the finding is that the occipital regions, which is typically associated with visual processes, showed modulated activities although the subjects were blindfolded. My colleagues in Berlin has already published dozens of navigation related studies and suggested that retrosplenial cortex, which is right under the posterior cingulate cortex, is involved in this process. Generally speaking, that could be the truth of functional brain mapping. However, when it comes to detecting retrosplenial cortex with scalp EEG recording, it sounds too ambitious for me. I found in literature that lingual gyrus is a posterior part of parahippocampal place area (PPA), which seems to fit as the explanation of the result I got from the study. So I put more weights on the lingual gyrus in the interpretation.
As an experimental approach in EEG analysis, I used group-level source information flow toolbox, groupSIFT, to calculate effective connectivity across probabilistic independent component densities resolved in the brain regions at the group-level analysis. Not surprisingly, I found time-evolving effective connectivity networks centered at the occipital and inferior parietal regions.
This paper has 25 pages, 12000 words, and 14 figures (plus 3 additional figures in the Supplement). I told grad students and post-docs that they should not write a paper like this because it risks their careers. The experimental paradigm is new, the analysis is new, no psychologically clever top-down question is available, etc. In a sense, it was good that I took this job because I was old enough.